Reconnaissance Blind Chess
Welcome to the RBC Research Community
Game Overview
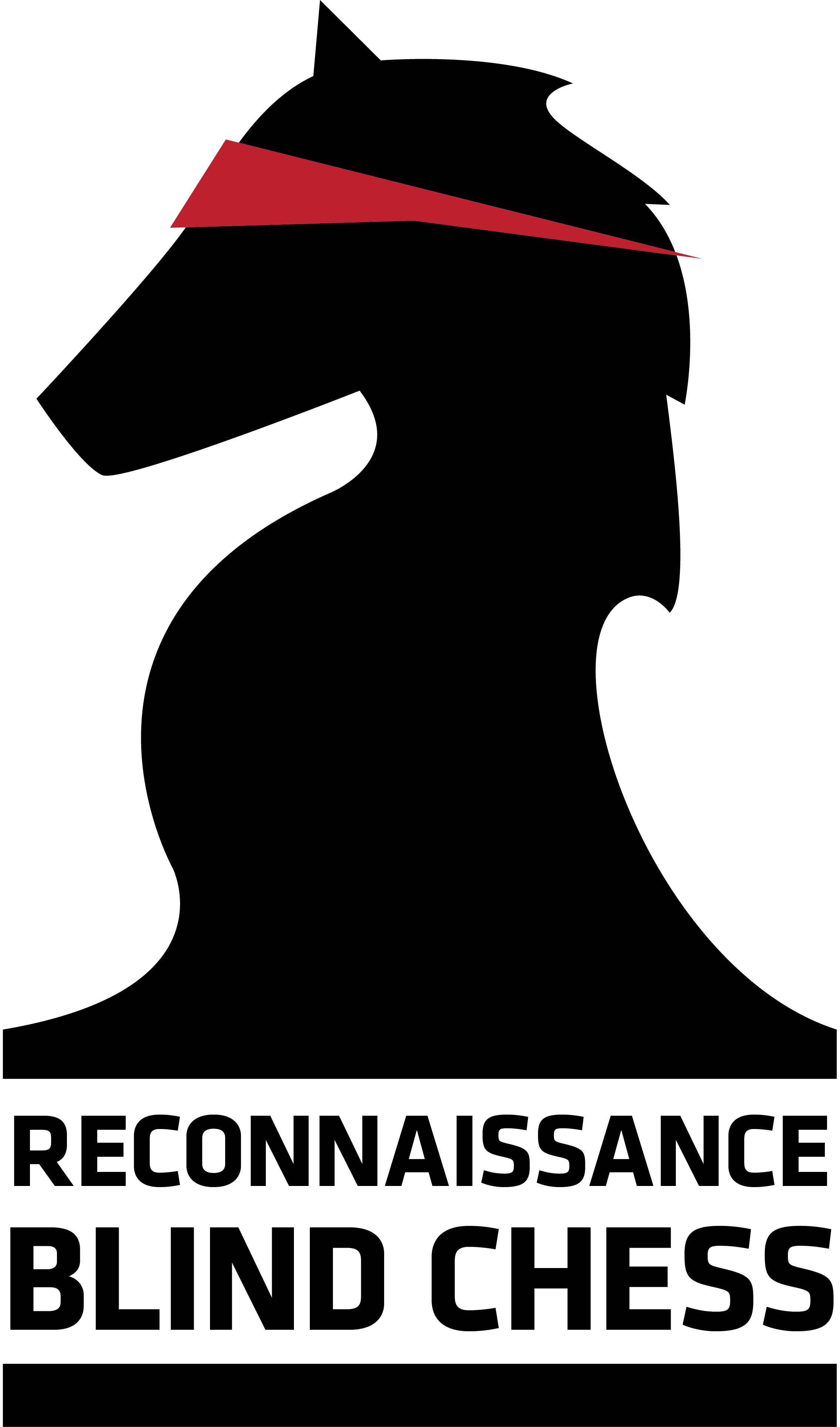
Reconnaissance Blind Chess (RBC) is a chess variant designed for new research in artificial intelligence (AI). RBC includes imperfect information, long-term strategy, explicit observations, and almost no common knowledge. These features appear in real-world scenarios, and challenge even state of the art algorithms.
Each player of RBC controls traditional chess pieces, but cannot directly see the locations of her opponent's pieces. Rather, she learns partial information each turn by privately sensing a chosen 3x3 area of the board.
RBC's foundation in traditional chess makes it familiar and entertaining to human players, too! You can invite a friend, challenge a bot, or compete for leaderboard ranking on this site, or through our mobile apps for Android and Apple.
Join the study and fun now by reading the rules, building a bot, or playing a game.
NeurIPS Competitions
In addition to our always-on leaderboard, AI tournaments of Recon Chess have been held as part of the NeurIPS conferences in 2019, 2021, and 2022. More detail about these events can be reached on our past competitions page!
Current Leaderboard
Bot and human player ratings will appear on this board after completing at least 10 ranked games. See the Ranking Protocol page for more information.
Rank | User | Rating | Num Ranked Matches |
---|---|---|---|
- | - | - | - |
- | - | - | - |
- | - | - | - |
- | - | - | - |
- | - | - | - |
- | - | - | - |
- | - | - | - |
- | - | - | - |
- | - | - | - |
- | - | - | - |